This year’s ISPOR – some reflections on AI
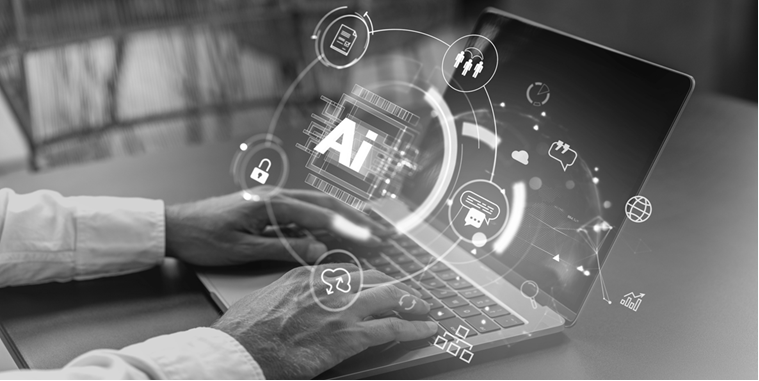
In our latest Insight, Managing Director Peter Lindgren reflects on AI and health economics.
There was no doubt about what was one of the big themes of the year at this year’s ISPOR meeting in Barcelona: AI. Although none of the plenary sessions were about exactly this, there were more than ten sessions on AI at the parallel sessions. In addition to this, several sponsored talks were held on the topic and there were also a bunch of posters. What can you take away from this?
To begin with, the spotlight is entirely on what is called generative AI (or large language models: LLM). Most people have probably at least played a little with models like ChatGPt or Gemini by now. However, the field of machine learning is larger than that, and the medical applications that exist make use of other techniques (here, for example, is an economic evaluation of one in sepsis).
In general, it can be said that the degree of concretization was quite low and, as often when AI is discussed, it is dominated by thoughts about what could be, but little about how (and if you are a bit mean, by consultants who want to profile themselves in the field). NICE’s newly released description of how they intend to continue working on AI issues received some attention, although in terms of content, it mostly says that they will continue the work. (Earlier this year, NICE released a recommendation on evidence generation using AI, which can be summarized as “be very careful, and ask us first”). So where do we stand practically today?
My assessment is that there are currently two areas where generative AI is of practical help to the health economist, and this was not affected in any direction by what was discussed at ISPOR. The first is, perhaps not surprisingly given what these models originally developed, is summarization and extraction of data from text and similar support in handling texts. (Depending on the language you are reading this in, there is a high probability that I used an LLM to quickly translate the text). No, ChatGPT will not write your GVD for you, but you can get good help with, for example, getting a 300-word summary. You do best to read it afterwards though. Most people are familiar with the tendency of language models to sometimes give incorrect answers, often described as the model hallucinating. Townsen Hicks, Humphries and Slater argue that one should instead say that it is bullshitting. The reason is that an LLM like ChatGPT has no idea of what is true or not, and it has no ability to reason. It only gives an answer that is likely given the input it has received. (For this reason, I’m less impressed when I see headlines where a model has performed better than humans on some test in the medical field: the fact that the model makes eight out of ten diagnoses correctly when a human gets seven out of ten right is probably compensated by the fact that the person knows when they are unsure and need to get input from a colleague. This is on top of the fact that the questions were probably included in the training data used when the model was created, which makes this type of test a pretty poor benchmark for model performance in itself). Hallucinations (or bullshit) make it important that a person is in the loop. An example of a very feasible application of this principle would be to replace a reviewer of which abstracts should be included in a systematic review with a language model. There is potential in being able to automate processes where large materials are reviewed automatically and where it is not practical with careful human control, but the gain must then be weighed against the fact that there will be errors in the data. How sensitive we are to errors is situation-dependent. One can easily imagine an AI that goes through medical records to see which patients are candidates to be included in a study: false positives will still be sorted out later and false negatives may not be the whole world. This appears fine, but the idea is to use the data for some kind of analysis, the situation immediately becomes more difficult due to biases that can creep in.
Another area where the models are helpful is in programming, especially for a person like me who once programmed a lot but is now a bit rusty to say the least. AI is a good help in finding solutions I know should exist, and which I otherwise might have spent more time trying to find an answer to stack exchange. Anecdotally, acquaintances who are really good developers are less impressed – for them, it is still faster to write code all by themselves. It may be that the benefits here are greatest for those who are in the middle of the distribution of skills – for the newest ones, the wrong solutions proposed by the model will take some time to figure out. It may be worth clarifying here that the help you can get is about developing a shell for a function that does something specific that you can then modify – we are very far from telling the model to program a Markov model according to a certain specification, even if there are those who have tried.
As with any field where there’s a significant amount of hype, it might be worth remembering that if it sounds too good to be true, it probably is – at least in the near future. However, development is advancing rapidly and if the problems of and bias can be solved, very useful tools may be at the door.
Författare
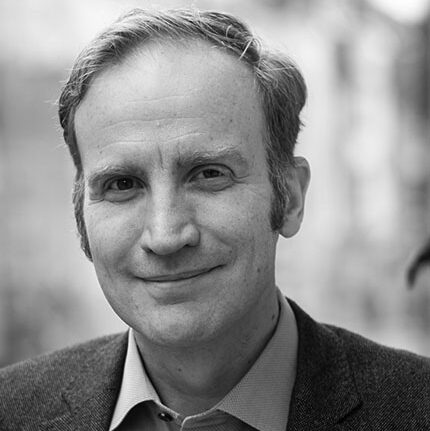